Delving into the dynamic realm of generative AI unveils significant developments in big-tech innovations and forecasts the trajectory of this rapidly evolving field. Over the last year, generative artificial intelligence (AI) has garnered substantial attention within the global tech sphere.
This surge in interest can be attributed to its groundbreaking capacity to revolutionize problem-solving, creativity, and decision-making for both businesses and individuals. The adaptability and efficacy of generative AI applications have prompted widespread adoption across diverse industries, from healthcare to entertainment, resulting in a notable expansion of its market size.
As of 2023, the global generative AI market reached a valuation of $12.1 billion. Projections indicate a remarkable ascent, with expectations that this figure will soar to $119.7 billion by 2032.
Furthermore, in 2022, a period predating the mainstream recognition of generative AI, startups in this domain successfully secured $2.6 billion across 110 deals. The landscape evolved rapidly, with funding skyrocketing to nearly $50 billion in 2023. Leading companies such as OpenAI, Anthropic, and Inflection AI played a pivotal role in this surge, each securing several billion dollars.
The escalating interest in generative AI is evident in the surge of searches related to the term. The chart below illustrates a pronounced spike in interest, particularly after the release of OpenAI’s ChatGPT platform, reaching its peak in June. Countries such as Singapore, China, Hong Kong, India, and Israel stand out as hubs where interest in this technology is flourishing.
As the landscape of AI-driven technology undergoes continuous transformation, its application extends, prompting a growing number of companies to incorporate these technologies into their business operations.
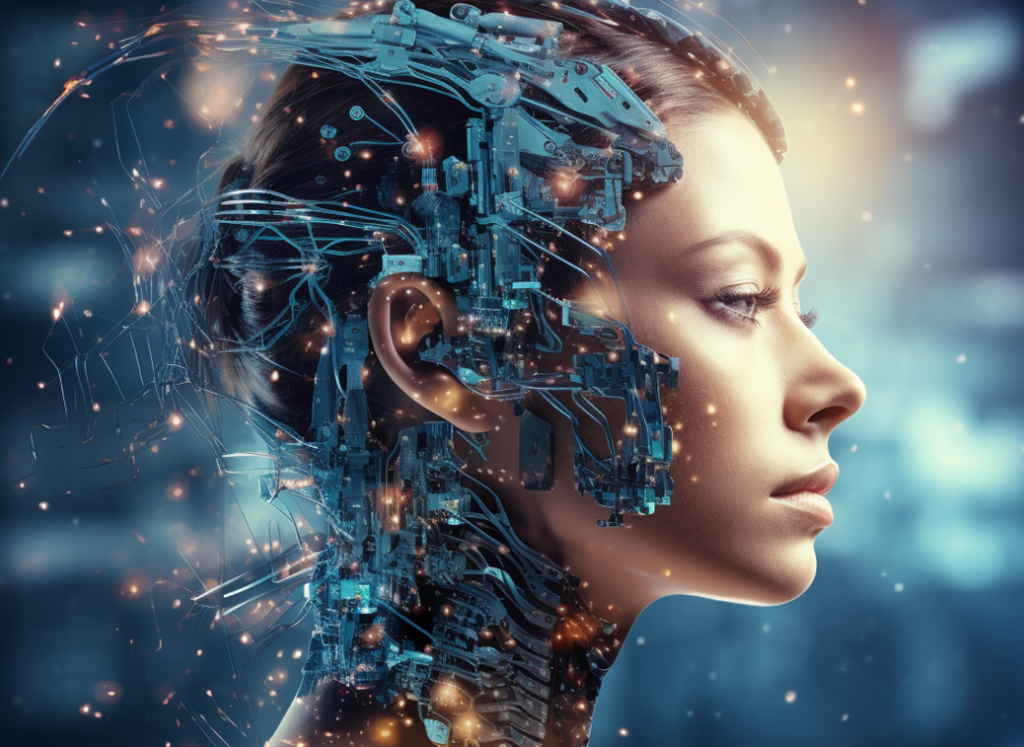
Big players delving into generative AI.
Ilan Rakhmanov, the founder and CEO of ChainGPT, an AI infrastructure provider catering to blockchain entities and Web3 projects, emphasized the increasing accessibility of generative AI for well-established brands. He noted that many such brands now view generative AI as a competitive advantage. Despite our current understanding of its capabilities, there remains uncertainty about its long-term evolution. This uncertainty stems from the expanding utilization of the technology by organizations and individuals, coupled with an increasing number of models training on associated datasets.
Noteworthy developments in mainstream adoption of generative AI include JPMorgan’s recent announcement of DocLLM, a generative large language model designed for multimodal document understanding. This model claims the ability to analyze and process diverse enterprise documents, ranging from forms and invoices to contracts and reports, which often feature intricate combinations of text and layout.
DocLLM distinguishes itself through its distinctive operational framework, diverging from the prevalent reliance on image encoders seen in other multimodal language models. Instead, it prioritizes bounding box information, enhancing the integration of spatial layout structures. This is achieved through an innovative disentangled spatial attention mechanism that optimizes the attention process in traditional transformers.
Amazon has also enhanced its generative AI capabilities by introducing a new tool to aid sellers on its platform. This tool generates precise and compelling product descriptions, significantly simplifying the listing process. It has already gained popularity among the majority of Amazon sellers.
Mistral’s latest Sparse Mixture of Experts (SMoE) model has garnered significant attention in the developer community due to its speed, efficiency, and extensive feature set. Being open-source, it has become a preferred tool for developers creating unique language models with limited resources.
DeepMind, a subsidiary of Google, remains a key player in the generative AI field, showcasing advancements in services like Google Brain and Google Translate. A recent noteworthy contribution is the introduction of Bard AI, a chatbot with capabilities mirroring ChatGPT, enabling users to generate high-quality text and creative content.
Amazon Web Services (AWS) has left an impact with the unveiling of Bedrock, a service granting access to diverse models from various AI companies. Bedrock stands out for its inclusive developer toolsets crucial for constructing and expanding generative AI applications.Salesforce, a cloud-based software company, has incorporated generative AI algorithms, collectively known as “Einstein GPT,” into its customer relation management platform. This integration significantly elevates customer engagement and personalization. IBM introduced the Watson AI platform, merging generative AI techniques with natural language processing (NLP) and machine learning (ML).
What can we expect in the future of generative AI?
Looking ahead, the future of generative AI appears poised for transformative growth, yet it faces challenges in an uncharted landscape. According to Rakhmonov, the trajectory relies on developing reliable models that deliver tangible value. The uncertain nature of generative AI, evolving with wider adoption and more data, raises concerns about the “black-box” nature of many AI models, potentially impacting public support.
Scott Dykstra, Chief Technical Officer and co-founder of Space and Time, emphasizes that despite the fervor around generative AI, the reality is nuanced. Generative AI’s future hinges on addressing challenges, such as the need for transparency in how AI models produce outputs, to maintain mainstream support.
Dykstra noted that, at present, most Fortune 500 companies are taking a cautious approach in the generative AI realm. This cautiousness is evident as many are content with merely incorporating an AI chatbot onto their websites without further exploration. He emphasized the challenge faced by enterprises operating at a large scale, citing the high costs associated with leveraging GPT-4 for enterprise production-grade products. He emphasized the need for reduced token prices, faster inference, and additional tools for automating retrieval augmented generation across the industry.
Challenges hindering the progress of generative AI.
Obstacles hindering the advancement of generative AI have been highlighted, with Dykstra pinpointing the speed of token streams as a critical technical challenge for models like LLMs. Achieving sub-second inference speed for a genuine LLM-based internet is an exceptionally challenging task, he explained.
In terms of development, Dykstra observed progress in AI-driven coding tools but noted that a breakthrough in “no-code” solutions is still pending. No-code solutions, requiring minimal programming skills for rapid application development, remain unresolved due to the intricacies of contextualizing entire codebases.
Rakhmanov’s focus is on the broader landscape influencing generative AI, particularly regulatory actions by governments. He considers these actions pivotal in defining acceptable AI practices. Additionally, he anticipates a potential global race for AI dominance, emphasizing the role of major tech players and countries like the United States and China. Critical discussions around computing power and chip production, according to Rakhmanov, will significantly shape the future of AI.
As we move towards a future shaped by technologies like AI, ML, and NLP, the evolution of the global digital landscape in the coming decade is poised to be intriguing.